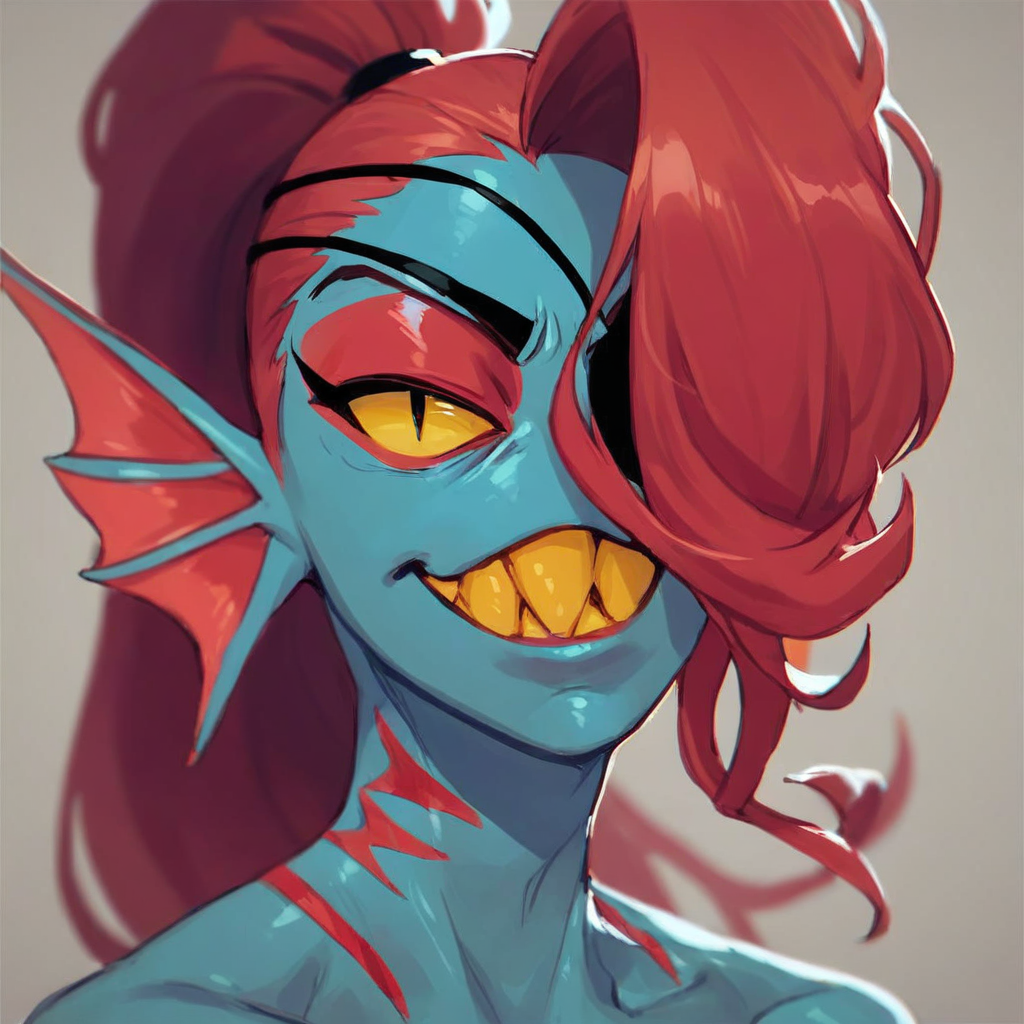
The Story of Pony Diffusion’s score_9 Image Quality Tags
VFX Pro ·When it comes to creating stunning digital art with AI, the secret often lies in the details. One of those details—known as score_9
—has been quietly transforming how AI models like Pony Diffusion create images. But what exactly is it, and why does it matter? Let’s dive into the fascinating journey of teaching AI to recognize beauty.
How AI Learns to See the World
AI image generation starts with two key phases: training and inference. Imagine training as teaching a student. The model learns from countless pairs of images and descriptions, trying to grasp concepts like “a cute pony” or “a sunset.” This process, which can take months, equips the AI to understand and replicate patterns.
Inference is the next step, where the trained model puts its learning into practice. This is like giving the student a test: the AI uses what it knows to generate new images. But just like students, AI isn’t perfect—it needs guidance to consistently create high-quality results.
Why Not All Data is Good Data
Here’s the catch: AI only learns from what it sees. If you train it with subpar or mismatched data, you get mediocre results. Yet, finding high-quality data is tricky. Not every concept (like cartoon-style ponies) has enough polished examples available, and it’s hard to draw the line between “good” and “bad” data.
To strike a balance, researchers must work with a mix of data, training the AI to distinguish between quality levels. Enter score_9
, a clever solution to this challenge.
Teaching AI to Recognize Quality
Score_9
is part of a system called aesthetic ranking, which helps the model identify what humans find visually appealing. At its core is CLIP, an AI model trained to link images and captions. By teaching CLIP about concepts like “masterpiece” or “best quality,” researchers can rank images based on their aesthetic appeal.
But CLIP isn’t perfect. While it excels at evaluating realistic or anime-style images, it struggles with less common styles like cartoons or ponies. To fill the gap, researchers manually labeled tens of thousands of images, rating their quality on a scale. This painstaking work created a dataset that helps Pony Diffusion generate better images.
The Power—and Pitfalls—of Tags
Using tags like score_9
in image captions allows the model to prioritize higher-quality images during training. This means that when you ask the AI for an image, it can focus on generating something more polished.
However, the system wasn’t without flaws. In Pony Diffusion V6, a tagging misstep caused the AI to over-rely on specific strings of tags, leading to inconsistent results. While this didn’t ruin the model, it highlighted the importance of continuous improvement.
Why It Matters to You
For users, the score_9
tags offer more control over image quality. Some tools even add these tags automatically, making it easier to get impressive results. However, the system isn’t perfect, and experimenting without tags can sometimes yield surprising outcomes.
Looking ahead, Pony Diffusion V7 promises to refine these methods, addressing past mistakes and pushing the boundaries of AI-generated art.
Conclusion
The journey to teach AI what makes an image “good” is a story of trial, error, and innovation. With tools like score_9
, Pony Diffusion is leading the way, making AI-generated art not just possible but exceptional. Whether you’re an artist or a curious onlooker, this is just the beginning of what AI can achieve.